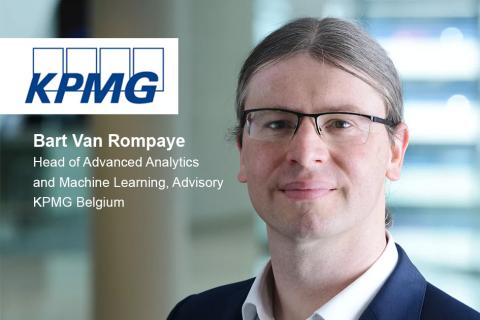
Published on 29 August 2023
Money laundering is the process of concealing or disguising the sources of illegally obtained money so that it appears to have come from a legitimate source. It is a global threat that has challenged banks for decades. The United Nations estimated the cost of global money laundering annually to be between USD 800 billion – USD 2 trillion, which makes up 2-5% of global GDP. Unfortunately, only 1% of the laundered money can be detected and acted upon by the authorities.
To increase the rate of detection, regulatory bodies are pushing the banks harder each year by updating anti-money laundering (AML) compliance requirements and tightening the controls. Most banks are still stuck in a traditional way of working, where the increasing regulatory demands translate into bigger teams focusing on money laundering problem, increasing the cost and the operational complexity, and often lowering the overall efficiency.
Banks provide the infrastructure that allows financial transactions to take place and as such, they also provide the first line of defense against money laundering activities of criminals and terrorists. Banks prevent these activities by implementing AML policies and procedures, verifying the customer’s identity using know-your-customer (KYC) processes, monitoring the transactions of the customers, reporting suspicious activities to authorities, and increasing the international cooperation to track offenders. Banks also train their employees to identify suspicious activity, implement new technologies for transaction monitoring and increase their cooperation with law enforcement. In recent years, the use of machine learning (ML) techniques has been gaining traction as a new way to combat money laundering.
This article focuses on the transaction monitoring aspect and will consider how banks are currently monitoring their customer’s transactions, as well as looking at how ML can be beneficial in detecting suspicious transactions for AML. Finally, we will also point out an increasing regulatory acceptance towards using ML.
Traditional way of “transaction monitoring” in banks
Transaction monitoring refers to the monitoring of customer transactions including assessing historical/current customer information and interactions to provide a complete picture of customer activity. This can include transfers, deposits, and withdrawals. Mostly using rule-based and scenario-based approaches, banks typically still have a set of pre-defined rules created by experts, which track and report suspicious activity to ensure that their customers are not engaging in money laundering activities. The rules and statistical thresholds associated with scenarios are driven primarily by industry red flags (like transactions to suspicious countries, transactions involving a shell company or foreign account), basic statistical indicators (like transactions over a certain amount, certain number of transactions) and expert judgment (e.g., where a banks' expert deems a certain specific sequence of transactions suspicious for a small enterprise). So, as a rule-based system relies on pre-determined criteria and set parameters, it is limited both by the level of insight of experts and the IT budget to implement these rules.
As the volume of transactions increases, the number of alerts increases, and furthermore the appropriateness of statistical thresholds starts to diminish. This increases the chance of false positives and false negatives, making it inefficient for banks to accurately detect potential money laundering activities. It is also important to increase the number of transactions in scope of a single assessment, as various means are used to hide financial crimes, such as ‘smurfing’ (spreading big money moves into multiple smaller transactions) or combining transactions in different currencies. Therefore, analyzing many transactions together will give a more comprehensive picture and help detect suspicious activities. However, this leads to highly complicated rule sets, with high chance of errors entering the code, and these are still limited in term of false positive rate. Combined with the high volume of transactions, the manual follow-up of the alerts is often time-consuming and labor-intensive.
While rule-based system designs may be the most straightforward way to translate concerns into an actionable system, they suffer from a host of downsides. Rule-based systems can be easily circumvented by money launderers who are familiar with the rules. These systems do not consider the full range of money laundering indications because they are programmed to respond to specific conditions. For example, they may be programmed to look for transactions above a certain amount, or transactions that involve countries known to have a high risk of money laundering. This means that they will not detect the more subtle indicators of money laundering, such as frequent small deposits and transfers, or transactions involving shell companies. Finally, rule-based systems require constant, explicit updates to keep up with the ever-changing patterns of money laundering, leading to high maintenance costs.
Inadequate control of money laundering activities may influence both the reputation and the financials of the bank. Reputational damage comes from banks facing criminal charges and customers may be less inclined to work with a bank that is not compliant with AML regulations. Financial damage may take the form of civil penalties, fines, and other sanctions if a bank is found to be involved in money laundering activities.
Machine Learning to the rescue
With machine learning (ML), powerful algorithms are used to extract patterns that are historically associated with suspicious activity. The ML approach to automated pattern identification leads to more precise and effective screening, allowing for more efficient use of the compliance team’s time. ML removes the need to manually define rules to sift through data. Our experience at a leading bank showed that their identification of suspicious activity can be improved by up to 40% by replacing rule-based and scenario-based tools with ML models.
Banks can choose to develop an ML model themselves that can be adapted to their specific needs. But sometimes organizations cannot build ML models themselves because of the cost and complexity of building such a model, the need for costly data sets, the expertise needed to train the model, and budget etc. In these cases, organizations can opt for vendor solutions that may provide greater cost-effectiveness, as they have already been developed and tested. Vendor solutions may also provide a wide range of services like transaction monitoring, customer due diligence, risk assessments, and other features and benefits that would not be available with a custom solution. There are more and more powerful AI-based AML solutions in the market. In one example, DISCAI (a fintech created by KBC bank, a midsized bank-insurer known for its digitality) brings an advanced AI-based AML tool to the market, which beats conventional rule-based tools used by most of the banks in terms of efficiency and effectiveness. This vendor solution uses highly advanced AI technology for finance, providing data analytics capabilities that enable banks to track and monitor transactions more effectively, reducing the chances of money laundering activities going unnoticed. Many vendor solutions not only give easy access to very advanced modeling, but very often follow a Software as a Service (SaaS) or AI as a Service (AIaaS) approach, also unburdening the organization from operational and IT concerns. At KPMG in Belgium, we are working with DISCAI, who provide just such an AIaaS solution, giving banks direct access to integrated screening of behavioral patterns, networks, and anomaly detection.
The recent advancements in applying machine learning to AML help alleviate the ever-increasing regulatory pressure on this topic. Financial institutions that do not change their screening paradigm, find that this regulatory pressure leads to ballooning compliance teams, with the growing cost and operational burden of screening. Changing to an ML paradigm can help organizations stop this evolution, as the gains in efficiency and effectiveness translate into smaller teams that can still respond to the ever-higher regulatory demands.
Regulatory acceptance
Banks have systems, controls, and policies in place to deal with AML regulation. These policies include procedures for identifying and reporting suspicious activity and for cooperation with law enforcement agencies. In recent years, regulators have not only put the bar for the effectiveness of these systems higher, but they are also frequently handing out higher fines for deficiencies in operations and non-compliance with regulations.
Recently ING was fined EUR 3 million for failing to comply with AML regulation. However, regulators are generally becoming more accepting of AI-based solutions as they become more sophisticated and accurate. As an example: the Dutch Central Bank (DNB) had originally prohibited Bunq's use of AI and machine learning to conduct AML monitoring - a move that led Bunq to sue the central bank, with the court subsequently siding with Bunq. Losing the lawsuit does not mean that regulators are more accepting, but it does confirm that regulators are expected to open up to new technologies being used to conduct AML monitoring, and that in the future they will be expected to seriously consider alternatives to traditional methods.
Conclusion
Banks have a complex and important role in the fight against money laundering. By implementing effective AML solutions, banks can help to make the global economy safer and more transparent. Clearly, breaking out of the traditional framework with ML can enhance operations. By streamlining the process of detecting unusual transactions or using vendor solutions, ML can help banks to better identify suspicious activity and help prevent money laundering. Vendor solutions are a great alternative where an organization does not want to develop their own machine learning models.
With the right implementation and execution, ML can be an effective way to combat money laundering and ensure that financial institutions are compliant with regulations.
Author: Sayali Kulkarni